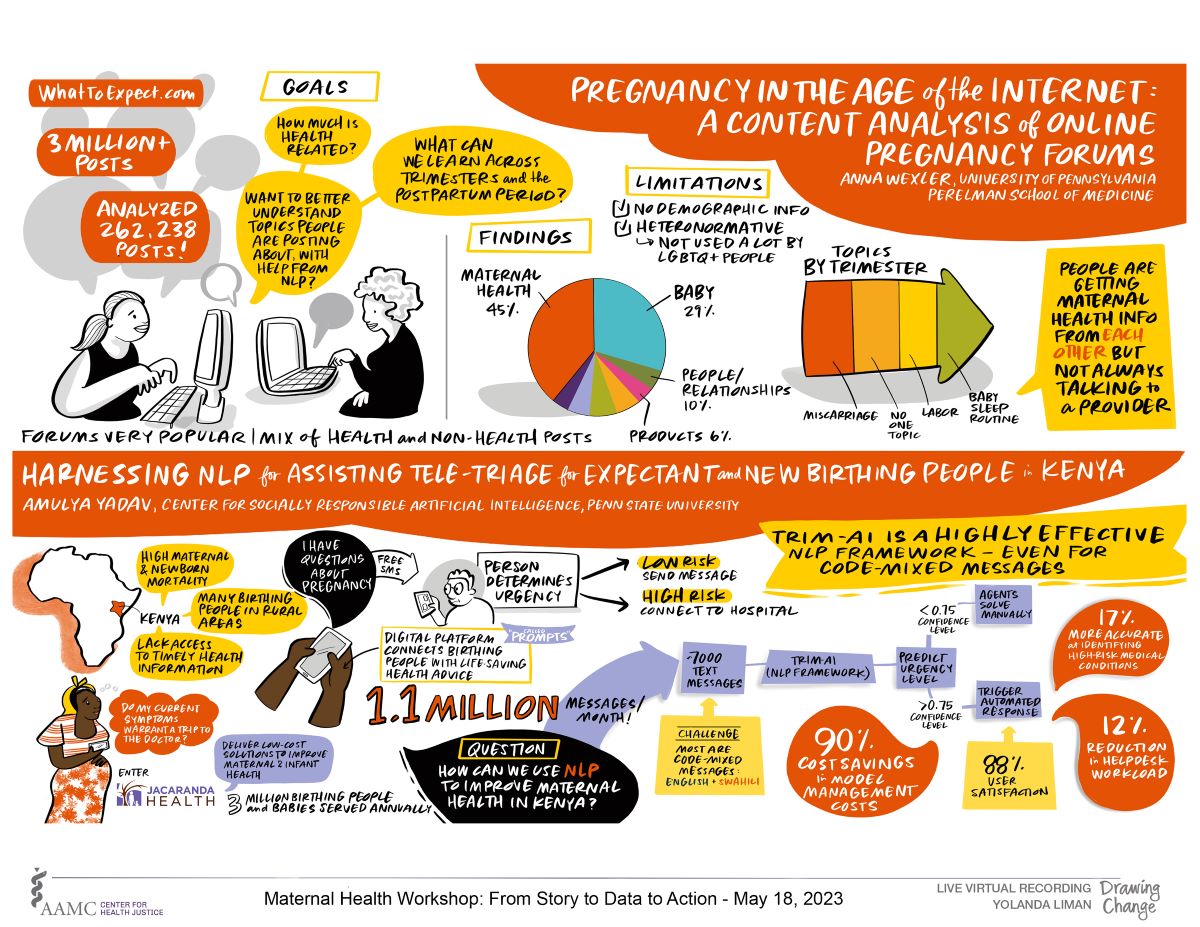
About this Graphic
This is a hand-illustrated graphic recording, created live during a keynote presentation at the Maternal Health Equity Workshop: From Story to Data to Action, May 18, 2023, which was convened by the Association of American Medical Colleges Center for Health Justice. Graphic recordings were created by Drawing Change. The effect is dynamic and organized into two main sections, top and bottom. There are limited illustrations in this piece and text is separated by background shading and different lettering sizes.
Pregnancy in the Age of the Internet: A Content Analysis of Online Pregnancy Forums
With Anna Wexler, University of Pennsylvania, Perelman School of Medicine
An illustration of two stylized figures with long curly hair looking for information online, with neutral facial expressions. Above them, bullet points about the website WhatToExpect.com explain: 3 million+ posts, analyzed 262,238 posts! The text below the figures says, “forums are very popular; a mix of health and non-health users.”
Goals
The next section is with a different background color. The goals are:
- How much is health related?
- Want to better understand topics people are posting about, with help from NLP
- What can we learn across trimesters and the postpartum period?
Findings
There is a brightly colored pie chart for this data with shading to make it look 3D.
- Maternal health, 45%
- Baby, 29%
- People/relationships, 10%
- Products, 6%
Limitations
No demographic information; heteronormative; not used by a lot of LGBTQ+ people.
Topics by trimester
A brightly colored arrow facing right, and the sections are from left to right:
- Miscarriage (left, in red)
- No one topic (next section, in orange)
- Labor (in yellow)
- Baby sleep routine (right, in green)
The final sentence of this presentation is in bold text on a bright background. "People are getting maternal health information from each other but not always talking to a provider."
Harnessing NLP for Assisting Tele-Triage for Expectant and New Birthing People in Kenya
With Amulya Yadav, Center for Socially Responsible Artificial Intelligence, Penn State University
Maternal Health Inequities in Kenya
An outline of the continent of Africa with Kenya highlighted with a star. Three circles around this read:
- High maternal and newborn mortality
- Many birthing people in rural areas
- Lack access to timely health information
Jacaranda Health
A Black Kenyan woman with a bright headscarf, wearing a printed skirt and short sleeves, is thinking “Do my current symptoms warrant a trip to the doctor?” Enter Jacaranda Health (with logo), which delivers low-cost solutions to improve maternal and infant health, serving 3 million birthing people and babies annually.
A pair of Black hands are texting on a cell phone “I have questions about pregnancy,” a free SMS. This is a digital platform that connects birthing people with life-saving health advice.
SMS Triage
The text goes to a person who reads it, a health care provider who determines urgency. Two possible next steps:
- Low risk, send message back; or
- High risk, connect to hospital.
Using NLP
Huge typeface: 1.1 million messages a month! The big question is how can we use NLP to improve maternal health in Kenya? A curving arrow connects to the flow of these steps:
- There are approximately 7,000 text messages (a challenge is that most are code mixed messages, English and Swahili);
- TRIM-AI (NLP framework)
- Predict urgency level: if <0.75 confidence level, then agents solve manually but if >0.75% confidence level, trigger an automated response.
Outcomes
TRIM-AI is a highly effective NLP framework, even for code-mixed messages. Four key statistics are highlighted at the end with brightly colored abstract shapes behind them:
- There is 88% user satisfaction
- 90% cost savings is model management costs
- 17% more accurate at identifying high risk medical conditions
- 12% reduction in helpdesk workload