Foundations of Responsible NLP Use for Maternal Health Equity
About the Foundations
By Maria Antoniak1, Aakanksha Naik2, Irene Y. Chen3, Lucy Lu Wang4, and Carla S. Alvarado5
These foundations were distilled from the input received during the AAMC Center for Health Justice Maternal Health Equity Workshop: From Story to Data to Action which took place May 18, 2023, and a complementary survey of women and birthing people. The information collected during the workshop’s exercise was elicited via a variety of mechanisms: audience interaction with the demonstration chatbot interface; a general survey of previous interactions with artificial intelligence (AI) and natural language processing (NLP) and sentiments about each; and the discussions during the workshop breakout sessions. In addition, the research team complemented the information collected during the workshop with a literature review and a survey of women and people who had given birth in the last five years to specifically elicit information from those with lived experience and their interaction with NLP and AI.
The foundations are presented in no particular order and are to be considered as a set to guide those who use NLP as a research tool and/or as part of clinical decision support.
Include the voices of birthing people.
When designing, implementing, and evaluating, include and weight heavily the voices of people most affected by your NLP tool. Center the principle of “nothing about us without us,” first popularized by disability activists. While the perspectives of NLP researchers and health care workers are also very important, the most affected group — birthing people — must also be included in the design of your NLP tools and studies. The voices of women and birthing people, especially from groups made marginalized, are often dismissed in health care, so aim to reduce barriers and distance between the birthing person and their care team.
What does that mean for my NLP tool/study?
- Include surveys and user studies throughout your research and design process.
- Where possible, include women and birthing people and health care professionals in your research team.
- Incorporate literature written by women and birthing people and learn from related work about the birthing experiences, plus perspectives and needs.
- This doesn’t mean placing all the responsibility on women or birthing people or designing only for the birthing person. Part of supporting maternal health equity is encouraging support from the birthing person’s partner, family, and health care team.
- Be gender inclusive. Don’t automatically predict the gender of study subjects and remember to include the concerns of trans people in your study design. Use gender inclusive language and follow the HCI Guidelines for Gender Equity and Inclusivity For insight into the birthing experiences of LGBTQIA+ community see here.
Hold onto the human: empathy, emotion, relationships, and complexity.
Maternal health revolves around meaningful events that can forge, strengthen, and test the human relationships between the birthing person, their child, their partner and family, their social network, and their health care team. We should build NLP tools that account for human elements like emotion and empathy, and recognizing that each birth is unique, and each woman and birthing person has an individual set of circumstances, experiences, and preferences. Personal stories and voices should be highlighted, not flattened; contradictions and outliers should be valued, not discarded.
What does that mean for my NLP tool/study?
- Look for outliers and value what they can teach you, rather than removing them.
- A one-size-fits-all approach is probably not appropriate. Research and applications of NLP should prioritize personalization and/or allow the tool to be tailored by the individual birthing person or health care worker.
- Don’t be afraid to approach subjective tasks, like predicting satisfaction in the birthing experience, or tasks where inter-annotator agreement might be low, like labeling emotion-support in online birthing communities. Sometimes the goal isn’t to solve a problem but to better illuminate it.
- Include qualitative methods in your study design, complementing quantitative models with focus groups, interviews, grounded theory, etc.
- Support the expression of feelings and empathy by everyone involved. Optimize for giving the care team more space and time for conversation and trust building.
Be aware of power dynamics in the care and research teams.
Maternal health care has a long and fraught history of shifting power dynamics in the care team. These historical shifts, like male OB/GYNs replacing midwives in the early 20th century, can lead to both good and bad effects for the birthing person. It’s likely that the introduction of NLP tools could further influence these dynamics, and so NLP practitioners should know this history and work to improve rather than exacerbate existing hierarchies. In particular, NLP users should be mindful of the impact of their tools on midwives, doulas, and other care workers whose placement is already precarious.
What does that mean for my NLP tool/study?
- Understand historical power shifts in the care team. Consider carefully who you expect to use your tool and who will be impacted (or potentially replaced) by your tool. What kinds of unintended shifts could occur?
- Consider these questions: Whose data and experiences are represented in the training of the tool? Who will provide predictions and advice? Who will receive predictions and advice? Who will have access to and control over the NLP tool? Are you concentrating more power within a single care team role?
- Reflect on your own position in the power hierarchy as an NLP user collaborating with health care workers and birthing people. Who is making decisions about the design and application of NLP methods to maternal health?
Always center the agency and autonomy of the birthing person.
Maternal health research and health care has an unfortunate history of abuse and disregard for the birthing person’s agency, and any research or tool-building for maternal health care must be conducted in awareness of this context. Just as health care workers should respect the bodily autonomy of birthing people, NLP systems should not be used to replace or circumvent the decision-making of birthing people. Any predictions should be transparently communicated, reversible, and allow for recourse. Respect the birthing person’s experience; don’t work from a perspective of skepticism about the birthing person’s ability to make decisions for themselves. Birthing people own and are in charge of their experience.
What does that mean for my NLP tool/study?
- Explore the construction of NLP tools that increase the agency available to the birthing person. For example, NLP tools might be used to provide birthing people with resources, explanations, descriptions of what to expect, or assistance for communicating with health care providers.
- If NLP tools are used to make or assist in clinical decisions, this should be disclosed to birthing people, and where possible, providers should obtain direct consent from birthing people.
- There should be a clear system for recourse if the birthing person is unhappy or confused about the use of an NLP system during their pregnancy or birthing process.
- Maintain privacy. Collect only necessary data and store the data securely. Avoid perpetuating the over-surveillance of birthing people.
Know the politics and implications of your measurements.
Reliable data can spur action, inspire the allocation of resources, and improve outcomes, but much maternal health data is still missing. NLP tools can help fill these gaps by extracting information from text in electronic health records, including unstructured clinical and non-clinical notes. NLP can also be used to predict missing clinical data, which is important, but we must strive to earn the trust of women and birthing individuals to share self-reported demographic data.
However, using NLP tools for data collection can have unintended consequences: (1) An overemphasis on data collection can draw resources away from building solutions; (2) Reproductive health is politicized, and your measurements may be used as evidence in support of unexpected agendas; (3) Focusing only on measuring problems can contribute to “deficit narratives” that blame communities for their own challenges.
What does that mean for my NLP tool/study?
- Clarify your research and/or impact goals.
- Consider the current allocation of resources and where help is most needed when choosing which problems to tackle. While additional data collection can be very useful, even for well-known problems, it can also draw resources away from other important problems.
- Think through possible narratives and take care about which data is collected, what measurements are produced, and how those measurements are communicated to the public.
- Know that your measurements and predictions could contain information that could be used to incriminate the birthing person in some places and situations. Similarly, the data could sometimes be used for unintended purposes, like personalized advertising and the setting of insurance reimbursement rates.
Optimize for outcomes that support the whole person.
Much existing NLP research for maternal health has focused on maternal mortality and postpartum depression but not on the many other challenges facing birthing people and their care teams. Maternal health also encompasses sexual health, family planning, pregnancy, the “fourth trimester” of postpartum health and recovery, breastfeeding, and more.
How we define success is important. NLP research, like most AI research, is centered on structured tasks with defined and accessible outcomes, like maternal mortality rates. Reducing maternal mortality rates is important, but so are other outcomes, such as achieving a positive birthing experience, and breastfeeding without stress. By extension, outcomes outside of health care bounds, such as overall well-being, and public and population health level outcomes, are also to be considered and designed for.
What does that mean for my NLP tool/study?
- Create new datasets with labels prioritizing other parts of maternal health. Consider who and what is not currently represented in existing datasets.
- Use social media and birthing person interviews to get a holistic overview of what outcomes birthing people care about.
- Run more long-term user studies to support a broader range of outcomes of interest.
- The outcome doesn't have to be a number; be open to more subjective measurements.
- Adoption of your tool is also an outcome, and you don't always have to move the needle on a traditional NLP metric.
Respect and support your data sources.
Much of birthing people’s knowledge about birthing comes from friends, family, and their community (both offline and online). Generations of birthing people have passed down oral stories, written books, and created huge amounts of online content about pregnancy, labor, and the postpartum period. NLP studies and tools benefit from these datasets, using them as training examples and extracting information from them. But the ownership of this data should be considered and these NLP studies and tools should be designed to avoid supplanting systems of support that are already thriving.
What does that mean for my NLP tool/study?
- Beware the “paradox of reuse” in which the creation of an automated tool removes incentives for people to continue creating the training data that powers that tool.
- Give credit to the data sources. Use proper attribution, as this not only respects people’s work but also builds trust from your users and supports auditing of your system.
- Where possible, provide back links that lead your users to re-engage with the data source (e.g., by sharing their own experience in an online birthing community).
Fight against inequity and protect all groups of birthing people.
The health care system often treats birthing people differently based on their race, class, gender, etc. Racism, biases, and other forms of discrimination can spread into your collected datasets, influence your design process, and flow downstream into the impacts of your NLP study or tool.
While these issues exist throughout and beyond health care, maternal health care is particularly exposed to inequitable treatment. As examples: (1) For many people, childbirth is their defining, most invasive, and riskiest experience with the health care system; (2) Numerous decision points require women and birthing persons and care teams to work together to identify a pathway unique to their circumstances; (3) Some of these decisions rely on individualized evaluations of the birthing person’s experiences by the care team (e.g., feeling pain, bonding with the baby).
These exposures open the doorway to disparate treatment and heighten the long-term impact of mistreatment.
What does that mean for my NLP tool/study?
- When modeling outcomes, consider both disparate impacts and disparate treatments. Sometimes people with the same complaint aren’t given the same treatment (e.g., pain management inequities). But in other cases, applying the same treatment across the board, without regard for an individual’s circumstances, is also an inequity.
- Remember that NLP models can mirror biases embedded in the data collection instrument and therefore in the training datasets — even worse, they can overrepresent those biases, exacerbating the impacts of existing biases.
- When building tools that communicate with women and birthing people (e.g., chatbots), consider a diversity of vocabularies, accents, and languages. An NLP tool must be fine-tuned to the place where it will be used for research or clinical decision support.
- Actively recruit birthing people from diverse demographic groups to help inform the training of your tool, to give feedback on your NLP tool once designed, and also to participate in your NLP study and interpret findings. There is no substitution method that can replace the unique experiences of the intersectional lives of women and birthing people.
Learn from maternal health traditions and communities.
When designing NLP studies and tools, you don’t need to start from scratch. Thousands of years of cultural traditions, health and health care practices, and tool development already exist, and more recently, grassroots communities on the internet have formed to help birthing people prepare for and work through their challenges and experiences. Learning from these traditions and communities can help you avoid repeating mistakes made by others.
What does that mean for my NLP tool/study?
- NLP is one tool in a big toolbox. An interdisciplinary team can help you avoid reinventing the wheel.
- Storytelling is an important way for communities and generations to collectively teach and learn about maternal health, as well as a way for the birthing person to process their experience. Consider how NLP tools can support and learn from storytelling rather than replacing this practice.
- Misinformation also exists in these datasets. Consider carefully how to balance handling misinformation — recognizing that the root cause is often mistrust in the health care system and your NLP study could contribute to that mistrust — while also valuing the expressions of personal experiences. The Principles of Trustworthiness is a resource for health care, public health, and other organizations as they work to demonstrate they are worthy of trust.
about the methods used to develop these foundations
1PhD, Young Investigator, Allen Institute for Artificial Intelligence, Seattle WA
2PhD, Research Scientist, Allen Institute for Artificial Intelligence, Seattle WA
3PhD, Assistant Professor, University of California, Berkeley and University of California, San Francisco
4PhD, Assistant Professor, University of Washington, Seattle, WA
5PhD, MPH, Director of Research, AAMC Center for Health Justice
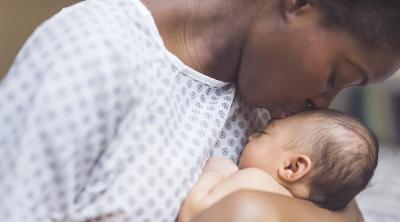
Maternal Health Equity
Maternal death rates and inequities in the United States are high — and on the rise. Learn more about the AAMC Center for Health Justice's maternal health equity work and resources.